4 min read
4 min read
MaRS & ThinkData Apply Machine Learning to Ontario Businesses
Tim Lysecki
February 25, 2020 7:00:00 PM EST
As we move forward in the data age, the companies that seek data are finding it. This is a good thing. There's undeniable value in using new sources of data, but before that value can be extracted, the various datasets coming from inside and outside any organization need to be able to refer to a given entity – a specific company, for example – in a standard way across the entire data catalogue.
It seems like a small obstacle; most humans can look at "GM" and "General Motors" and know they're related. But creating a scalable, programmatic solution for this is far more complex, and needs to account for every variation and permutation.
“It is extremely challenging to teach machines to understand how entities are represented and related to each other from disparate datasets,” says Hoyoung Jang, Lead Data Scientist for the DataLabs team at ThinkData Works, a company that specializes in data management technologies, specifically those addressing variety.
The problems in linking records aren’t unique to any one company. Especially when using alternative and external data, there is always disparity in the way that information gets recorded; each organization, department, and even person, has a different way of doing things. Siloed data, human error, and multiple sources of data are just a few ways that an address like “Acme Corporation” could be recorded as Acme Co., ACME CO INC, Acme Canada, AMCE Torotno, and so on. These kinds of incongruities often exist within one dataset, and are almost guaranteed to exist between two datasets, even if the source is the same. For this, data teams need entity resolution.
Each of these are derived from the same parent emoji, but are expressed in such different ways.
It's a problem of data variety
The team at MaRS Data Catalyst set out to create a holistic view of the landscape of start-ups and scale-ups; to do that, they needed to stitch together data from primary sources like data partners in the innovation economy ecosystem, surveys collected by MaRS, data providers like Crunchbase, and any tertiary data available. Essentially, every piece of data on a given company had to be tied to a single, unique identifier so as to be able to track changes to specific companies over time.
Especially with early-stage companies, there are so many changes that can take place: addresses, company names, product pivots, all of which need to be tracked over time and across multiple sources. It’s very messy, and it’s not a problem of handling 'big data,' it’s a problem of data variety.
“We had tried a few fairly standard Python libraries around entity matching. We also would take the list of names we had and simplify them, then compare those simplified versions.” Joseph Lalonde, Senior Data Manager for MaRS Data Catalyst, reflects on the manual processes they had in place. None of their solutions could keep up with the amount of data they wanted to flow into the solution, and that was holding the team back.
The MaRS team "would write rules to disambiguate companies, but inevitably, some get missed. These inaccuracies would get noticed, and they’d have to write a new rule and do it all again,” notes Zeshan Mahmood, Lead Software Developer on the DataLabs team at ThinkData Works. “Even with the great team they have, it’s incredibly time-consuming.”
A common thread through disparate data
ThinkData Works built a machine intelligent record linkage solution as part of our data enrichment services. A Toronto-based company, ThinkData is also part of the MaRS partner network and a MaRS IAF portfolio company – their data management solutions held the key to connecting the dots at Data Catalyst.
ThinkData's Jang points out one of the most complex pieces of the puzzle. “Most entity resolution solutions out there lack the ability to scale, which is key when you’re working with large data.” Creating a scalable solution to the problem of entity resolution was ThinkData's primary objective in creating er².
“We first try to classify type of entities that exist within the data. The benefit is three-fold: resolution is more consistent because it's given context; the classification helps to refine, enrich, and standardize the information for each entity type; and it provides meaningful entity relationships across datasets.”
For the Data Catalyst team, the outcome is faster, more reliable results that don't slow down with new data, all fully managed and backed by a team of dedicated data scientists and engineers.
“It saves us so much time having this solved by ThinkData's data engineers, and it’s definitely more involved in terms of the level of sophistication,” says Lalonde. “ThinkData Works has provided a visible improvement that we’ve seen over time. There’s also more of a feedback mechanism – a Python library will never have this responsiveness and level of customer service.”
Work smart, not hard
The team has been able to run entity resolution on their data without major disruptions to their workflow. Lalonde notes, “Because it’s programmatic, we can incorporate it into the rest of our data flows. It’s not some kind of tool that you have to jump in and out of to get it to work, it integrates with no friction.”
Using datasets coming from entirely different sources, the Data Catalyst team can now reliably tie entities together across hundreds of thousands of rows. With the entity matching piece solved, they're able to focus their time on using the data rather than wrangling it.
“It feels like it will get better and better, and it doesn’t seem to matter the number of companies we throw at it.” The benefits were immediate, and the payoff is only getting bigger.
“The more we use it, the more time it will save us.”
About MaRS Data Catalyst
MaRS Data Catalyst is combining analytics and rigorous research to promote socioeconomic impact. They help corporates, government agencies and academics share and use data through reports, insights and innovative products. From fostering data transparency to preparing citizens for the future of work, MaRS Data Catalyst believes that access to high-value information can make Ontario a better place to live, work and learn.
About ThinkData Works
Discover, govern, and monetize the data that powers your business. ThinkData Works offers a flexible enterprise data catalog designed to ensure data quality and regulatory compliance at every stage of the data lifecycle. To learn more, please visit ThinkData Works and connect with us on Twitter and LinkedIn.
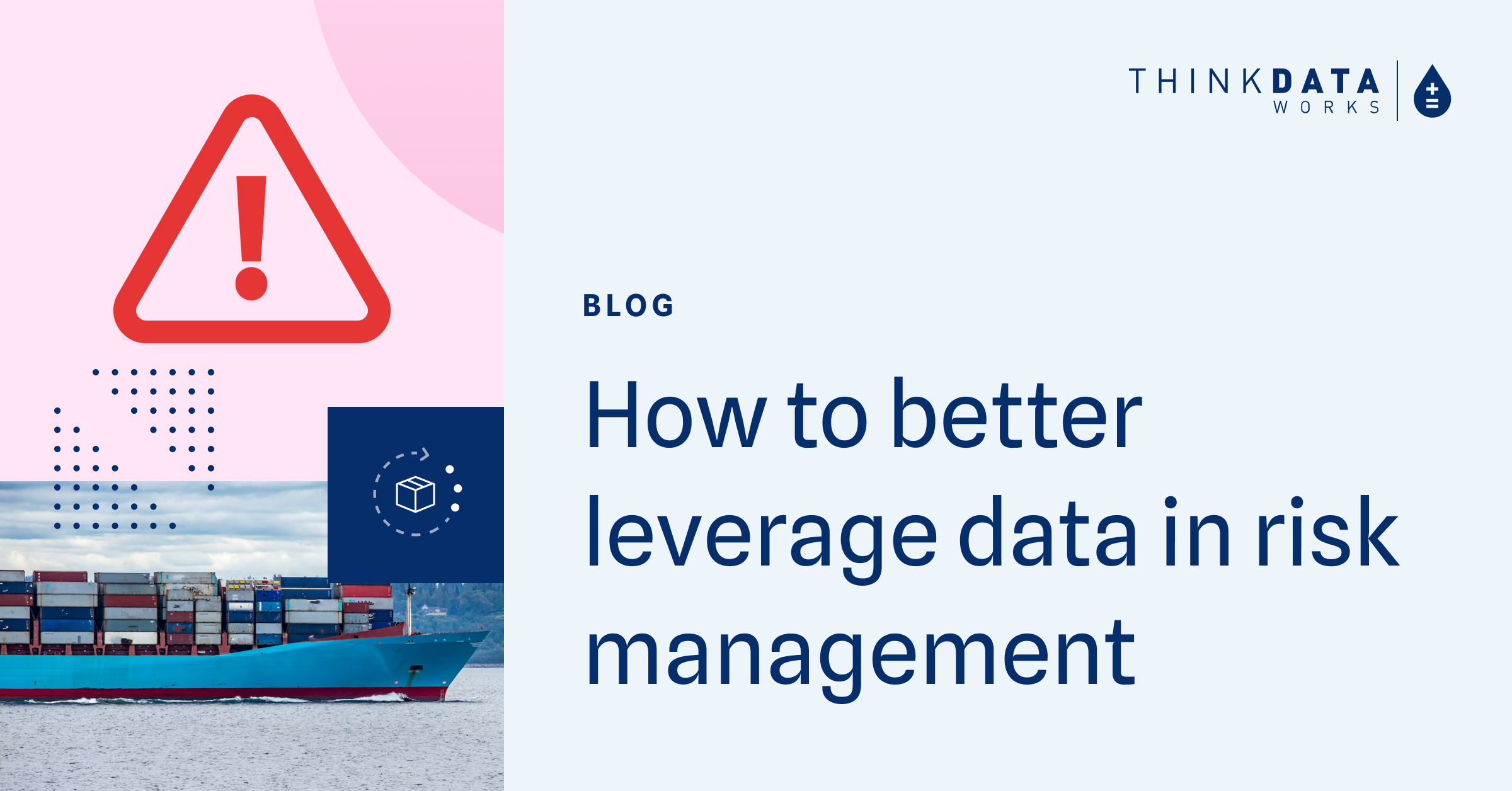
4 min read
How to better leverage data for risk management and crisis response
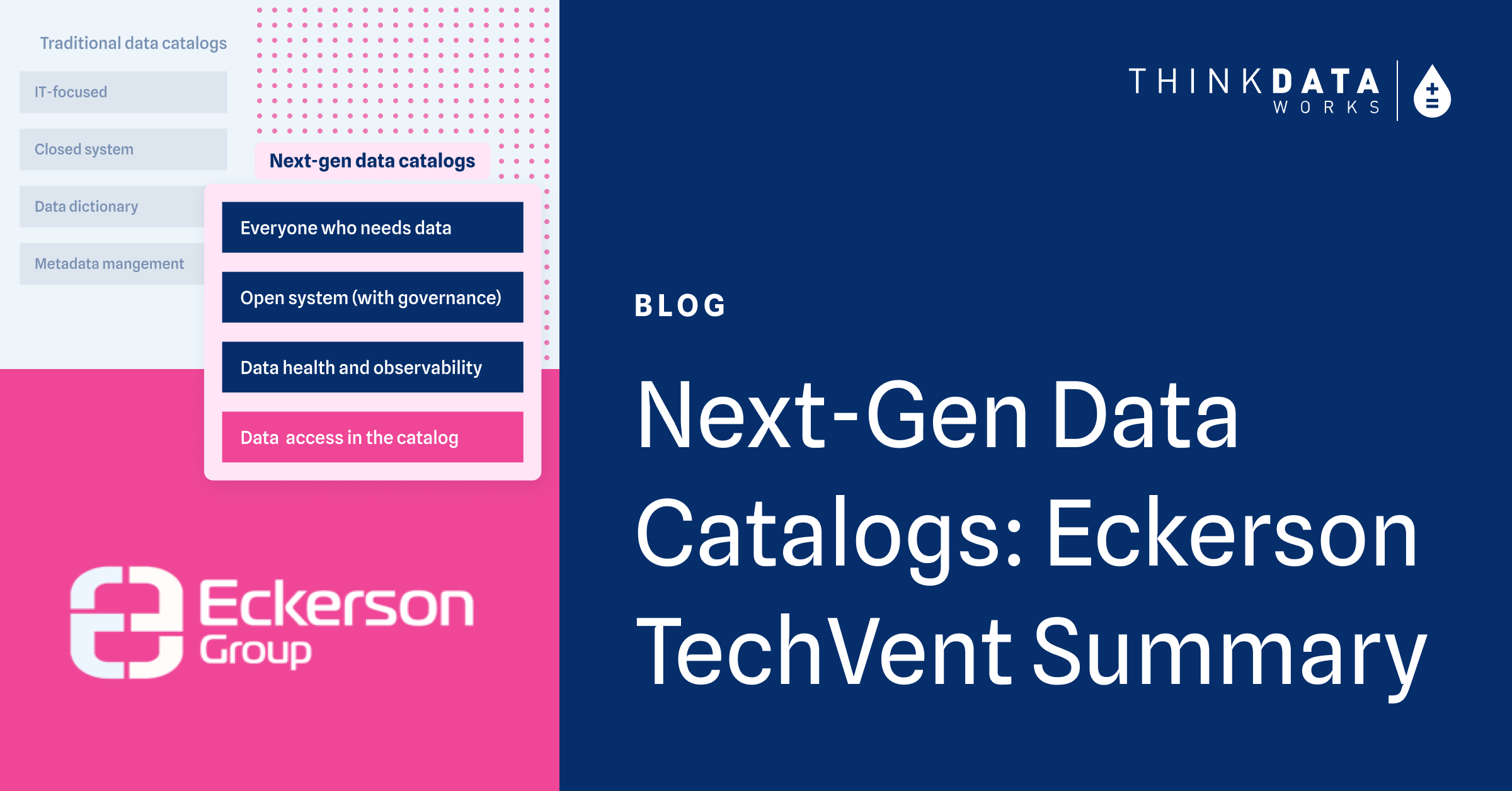
3 min read