4 min read
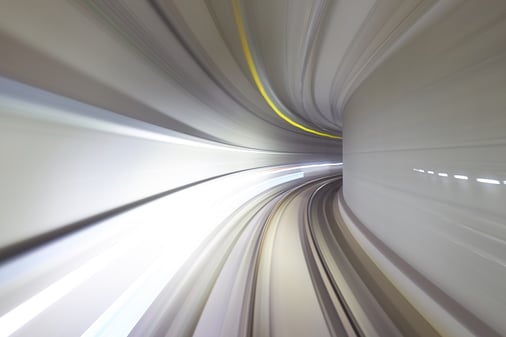
Is your data ready for AI?
For most companies, the answer is no.
Industry leaders in every sector are racing to adopt sophisticated systems that promise the introduction of AI and ML into their organizations. Executives often get sold on the concept of a technology that will give them the ability to see things that humans can’t, making suggestions or changes to business that can unlock new opportunities and drive major efficiencies.
Although the opportunities are definitely there, and in some cases so is the technology, there is a major disconnect between the possibilities that AI and ML are presenting compared to the operational readiness of most organization’s data environments.
“AI is like sex in high school. Everyone says they’re doing it, very few actually are, and those who are, are doing it wrong.”
The reality, though, is that a data warehouse and an application layer represent the beginning and end of a process, and what’s usually missing in the middle of this process is a pretty sophisticated stack that transforms raw data at one end, into usable information at the other. The problem is, not only is this “in-between” layer a difficult problem to solve, it’s also a major unknown for many decision makers setting IT strategy, budget, and timelines.
“If Watson can win Jeopardy, it must have the cognitive ability to wade through my data and develop insight, right?”
Often, organizations have a data warehousing strategy or a fully deployed data warehouse solution that focuses on getting data into one place. Appended to this strategy is usually a laundry list of already purchased applications or solution roadmaps using new technology (AI, ML, Automation, Analytics, Visualization). From a high level, it would seem as though the problem is solved:
Get all the data together — Check.
Purchase applications that turn raw data into insight — Check.
The concept here is that once we get all the data to a common place, we can plug it into whatever we want. Simple. The problem is, the data coming out of the warehouse(s) lack a common standard, and the applications sitting on top of the data can’t be fully implemented because…
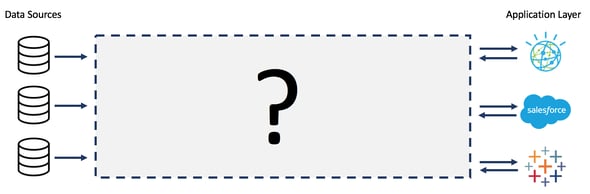
…there’s a massive refinement layer sitting in between the data (lake or no lake) and the enterprise apps that hasn’t been defined or implemented. To solve this problem, a system needs to be introduced that has the power to transform data from its native tongue into a centralized standard that any app can learn to understand. Not only that, but it has to be able to do this on a consistent and go forward basis and be able to scale to an infinite number of new and existing data sources.
Even the best software needs good data.
At the end of the day, any application, automation, or AI/ML technology needs to be fuelled by refined, standardized data in order to function to its greatest capacity. Without standard data, the game changing tech you wish to implement that promises to revolutionize your business will be limited to the lowest common denominator of standard data it can gain access to. Usually, that means an extremely limited amount of information even if it is now all coming from the same place.
Data needs to be refined into fuel and delivered in needed quantities to the applications it is tasked with powering. A system that does this, or better yet, a data refinery process that checks all of the required boxes, looks like this:
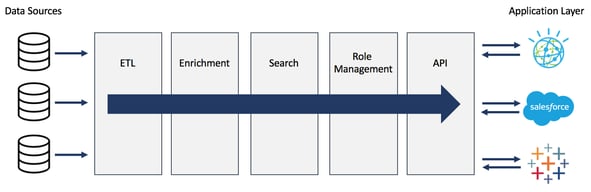
Or, for those interested in what we do for some of our clients with our platform, Namara, it looks like this:
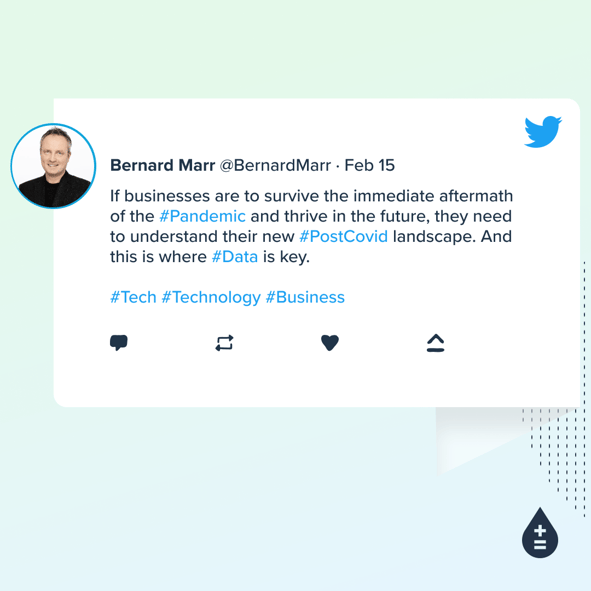
A lot of the really successful showcases of AI and ML technology is launched on the back of extremely clean and standardized libraries of data. But in most organizations, data is still quite segregated. Bringing data together into a “lake” or onto an enterprise cloud gets data in a common place, but it is often still lacking standardization among the data itself. This layer of data standardization is still a huge hurdle that many organizations have yet to get over. And the complexity of the problem only compounds with the addition of new, external data sources that forward thinking organizations are beginning to value and leverage.
A variety of data means a variety of problems.
The problem we’re trying to solve is that of data variety. Proper warehousing helps us solve volume and velocity issues, but a world with fully unlocked enterprise apps requires us to answer the question of how we transform similar data coming from multiple places into a common standard on a consistent basis.
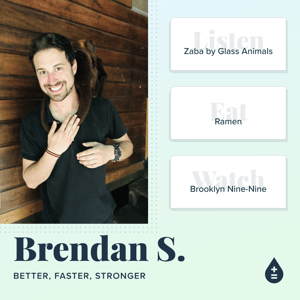
In many organizations, the answer is, “that’s the job of our data scientists”. But if we really think about it, should it be? Data scientists are highly skilled, well-paid individuals whose time and effort should be focused on helping the business grow in a data-driven way. If that’s the case, then why is so much of their time focused on finding, cleaning, standardizing, and monitoring data?
The short answer is because it has to be, given most organizations don’t have the right data processing pipeline in place to generate ideal data in a way that allows their apps and their scientists to work as efficiently as possible.
This turns most data scientists into data janitors, and it’s also is the reason most enterprise apps will never fully reach enterprise deployment.
“The problem isn’t lack of direction or ability. It’s lack of refined data.”
The idea of a data lake or cloud is a good one and a necessary concept. Data needs to be pooled into places so that it can be accessed by multiple functions for multiple reasons. A lot of good things became possible because people started realizing the benefit of knowing where their data was and what it was actually made of.
Now, as we look to the next phase of business intelligence, one that brings with it automation, artificial intelligence and machine learning, we must think less about data sitting in a lake, and more about how that data needs to flow throughout an organization in a standard way. We’re evolving beyond the need for a container that can hold our data and we’re moving towards the need for a vascular system that can flow data to where it needs to be in the shape and form that is required.
This may seem like a huge leap forward, but in reality, it’s very much attainable. In fact, many of the pieces required are laid out in the above graphics. On your own, it’s not a quick or easy build, but it is a necessary problem to solve if you are truly thinking from an enterprise perspective.
The takeaway here is not to stop looking at new technology that can help unlock huge potential. It’s to remember that regardless how shiny and amazing new solutions are, they all require rocket fuel to help them blast off. When we talk game changing enterprise apps, the rocket fuel is standardized data. And a process to refine it needs to be implemented in parallel with the adoption of new Business Intelligence tools and applications.
Read more about how we're delivering clean libraries of data to drive AI tech.
Want to learn more about aligning your business and data strategy?
Request a consultation with one of our data experts or browse the largest catalog of solution ready data to determine how ThinkData’s tech can advance your projects.
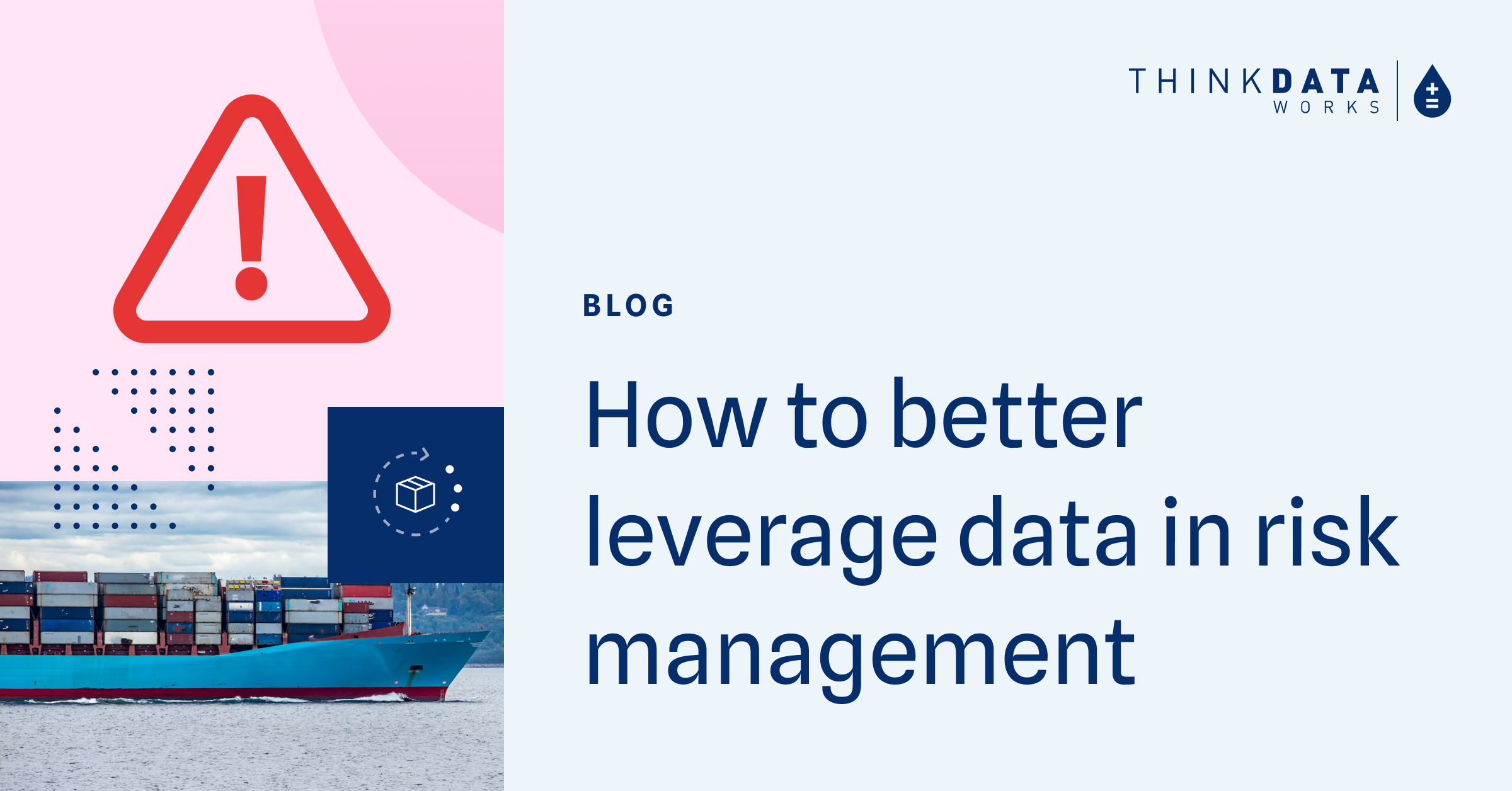
4 min read
How to better leverage data for risk management and crisis response
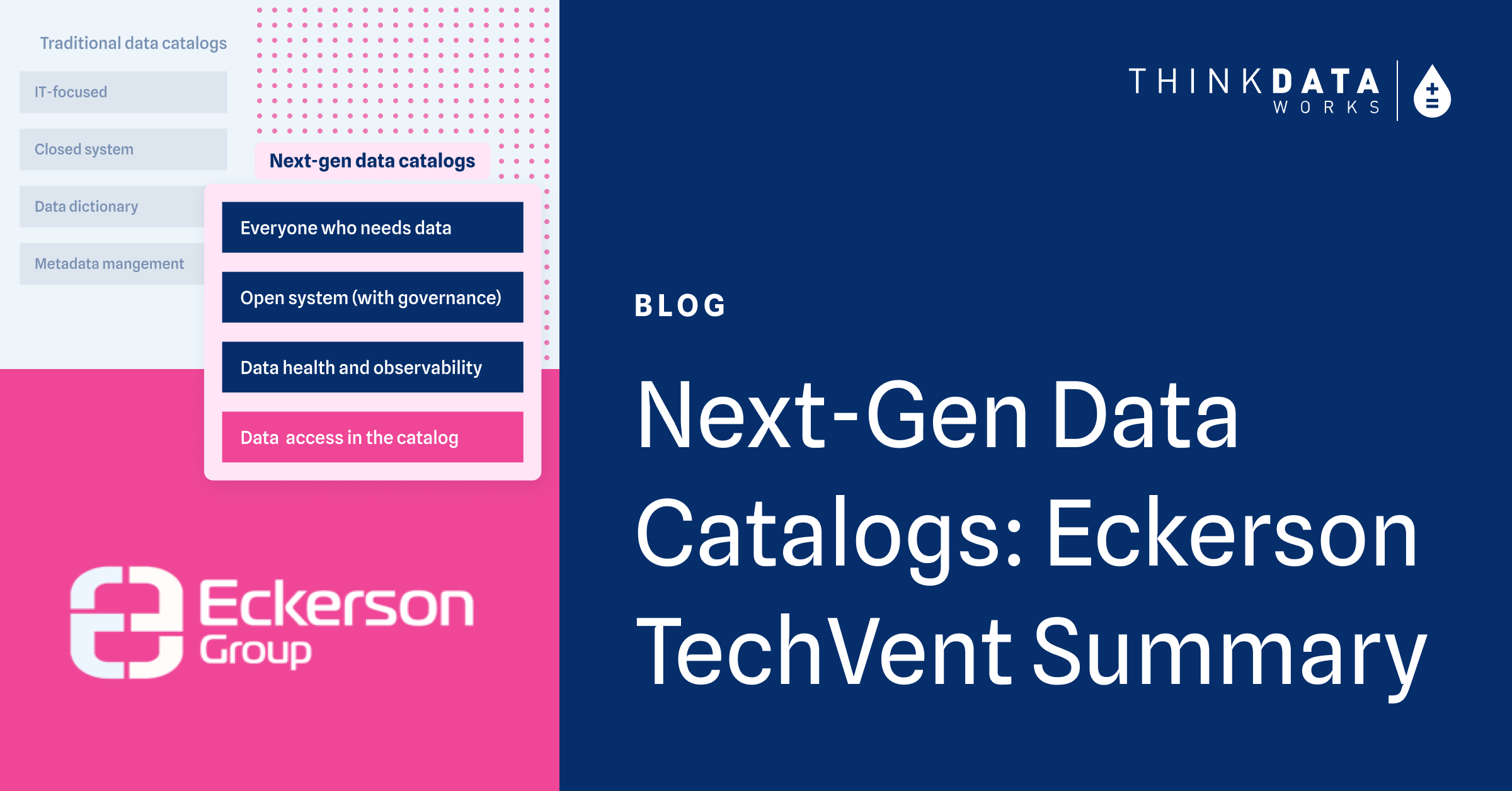
3 min read